The Power of AI in E-commerce: AI-Driven Strategies to Boost Customer Engagement and Business Growth
Discover AI’s power in e-commerce, enhancing customer engagement and business growth with advanced s
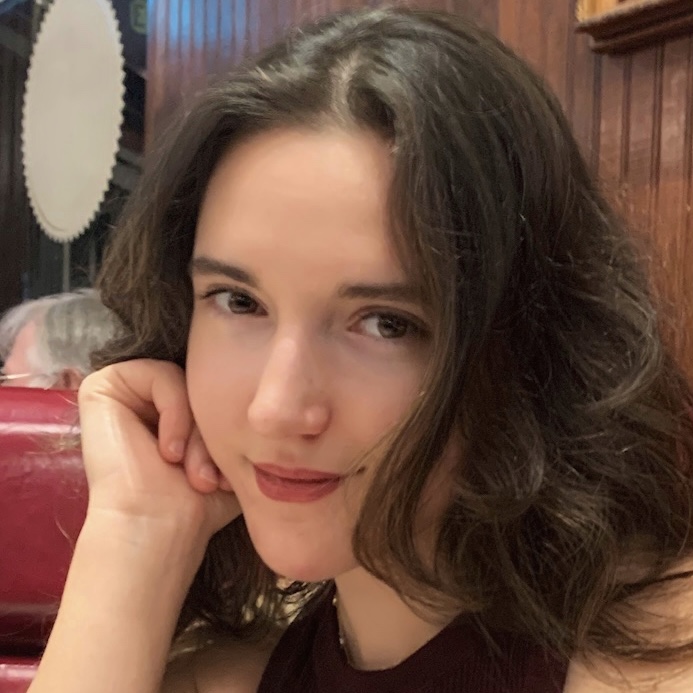
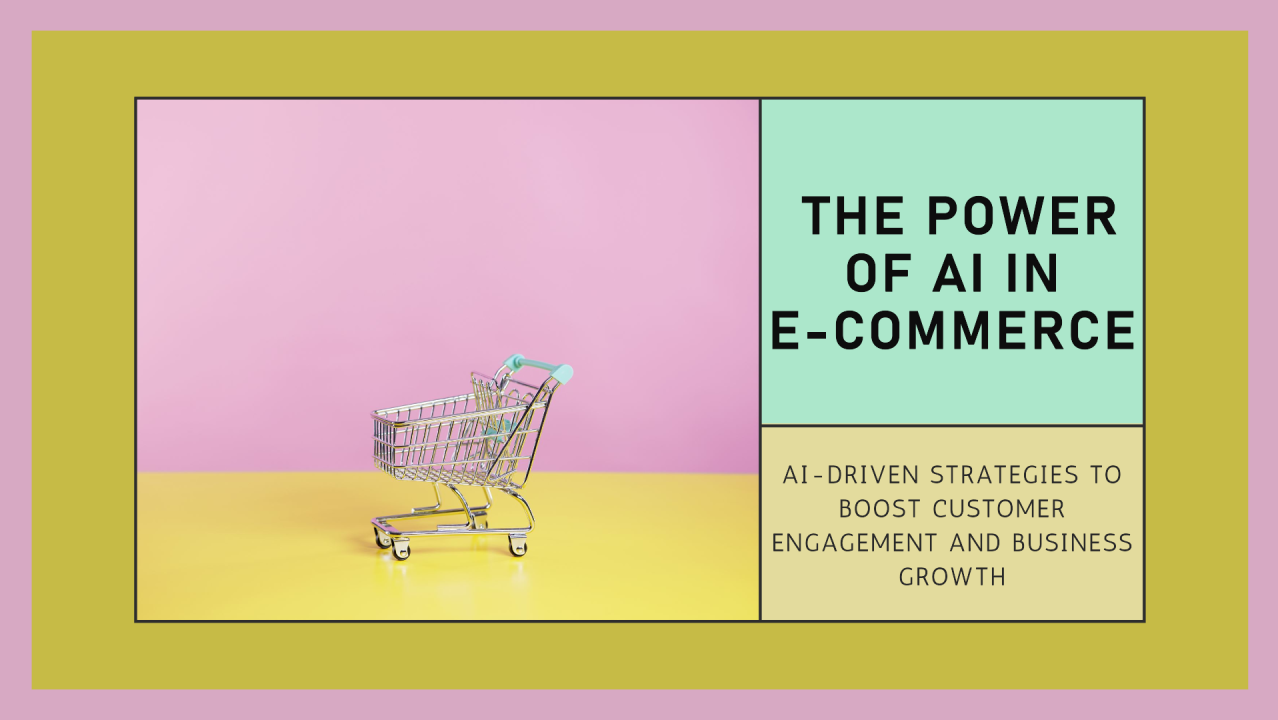
In their appearance at the Index Conference 2024, Luming Chen (Machine Learning Engineer) and Sudeep Das (Head of Machine Learning and AI) highlighted the innovative strategies DoorDash employs to enhance consumer engagement.
This article explores how cutting-edge machine learning techniques and recommendation systems are revolutionizing e-commerce, focusing on the approaches used by industry leaders like DoorDash.
What is Personalization in E-commerce?
Personalization in e-commerce goes beyond simply addressing customers by their first names in emails. It involves predicting consumer behavior to present the most relevant products and offers at the right time. This level of personalization can significantly increase customer satisfaction, loyalty, and conversion rates. Advanced algorithms achieve this by analyzing vast amounts of data to uncover patterns otherwise impossible to detect.
Machine Learning Algorithms: The Backbone of Personalization
Machine learning (ML) algorithms are the engines that drive personalization in e-commerce. These algorithms analyze user data, including browsing history, purchase history, and real-time interactions, to build detailed customer profiles. Here are some of the ML techniques used in e-commerce personalization:
1. Collaborative Filtering
Collaborative filtering is one of the most common recommendation techniques. It identifies similarities between users based on their behaviors and preferences, suggesting products that similar users have liked. Although effective, this approach can struggle with new users due to their little interaction data, a problem known as the “cold start.”
2. Content-Based Filtering
Content-based filtering recommends products based on the characteristics of items the user has shown interest in. To illustrate, if a user repeatedly buys coily hair shampoo, the system might recommend other haircare products for coily hair. This technique excels in scenarios where user data is sparse but relies heavily on detailed item descriptions.
3. Hybrid Models
Hybrid recommendation systems combine collaborative and content-based filtering to leverage the strengths of both methods while mitigating their weaknesses. By integrating multiple algorithms, these models can provide more accurate and diverse recommendations, enhancing the overall user experience.
4. Deep Learning
Deep learning recognizes complex patterns in data to produce accurate insights and predictions. Convolutional neural networks (CNNs) can analyze visual content, while recurrent neural networks (RNNs) can analyze sequential data. These methods excel at personalizing content in multimedia-rich environments like video streaming services.
Case Study: DoorDash's Personalization Strategy
DoorDash, a leader in food delivery, has expanded its mission to include groceries, convenience items, and retail products. To ensure a seamless consumer experience across these diverse product verticals, DoorDash employs sophisticated machine learning algorithms. Their strategy involves understanding each consumer's purchasing history, dietary restrictions, favorite brands, and other personalized details.
Implementing Two Tower Embeddings
DoorDash's recommendation system uses a technique known as two-tower embeddings. This method involves creating two separate embeddings: one for users and one for items. The system then calculates the similarity between these embeddings to generate personalized recommendations. This approach allows DoorDash to handle the complexity and scale of its extensive product offerings efficiently.
Leveraging Large Language Models (LLMs)
DoorDash incorporates large language models (LLMs) like GPT-4 to enhance further its recommendation system. LLMs can understand and generate human-like text, enabling more nuanced and contextually relevant suggestions. For instance, by analyzing customer reviews and feedback, LLMs can identify sentiment and preferences, refining the personalization process.
Embracing Causal Inference
Causal inference techniques help DoorDash determine the impact of specific actions on customer behavior. By understanding the cause-and-effect relationships between different variables, DoorDash can optimize its recommendations to drive desired outcomes, such as higher order values or increased frequency of purchases.
Profiting from Advanced Personalization
Increased Customer Engagement
Presenting products that match the customer's interests and needs
leads to higher interaction rates and longer browsing sessions. Ultimately, personalized recommendations generate more sales.
Enhanced Customer Loyalty
When customers feel understood, they have a better experience overall, meaning they are more likely to return to the platform. It works because it taps into the most raw element of brand loyalty: emotional connection. Hence, personalized experiences produce repeat business.
Improved Conversion Rates
Relevant recommendations reduce time spent searching for products, streamlining the purchase process. This convenience translates to higher conversion rates and increased revenue.
Efficient Inventory Management
Advanced algorithms can also help e-commerce platforms manage their inventory more effectively. By predicting demand for specific products, companies can optimize stock levels, reduce waste, and improve supply chain efficiency.
Conclusion
As competition intensifies online, companies are turning to advanced algorithms to deliver tailored experiences that resonate with individual customers.
Advanced personalization is transforming the e-commerce landscape, with companies like DoorDash using machine learning. As these technologies evolve, we can anticipate even more refined personalization in e-commerce. Thus, understanding these advanced algorithms and their applications is integral to enduring competition.
Product managers can drive impactful changes that enhance customer satisfaction and business performance by focusing on customer engagement strategies and leveraging cutting-edge technologies.